THE IMPORTANCE OF ULTRA-LOW LATENCY EDGE INFERENCING FOR REAL-TIME AI INSIGHTS
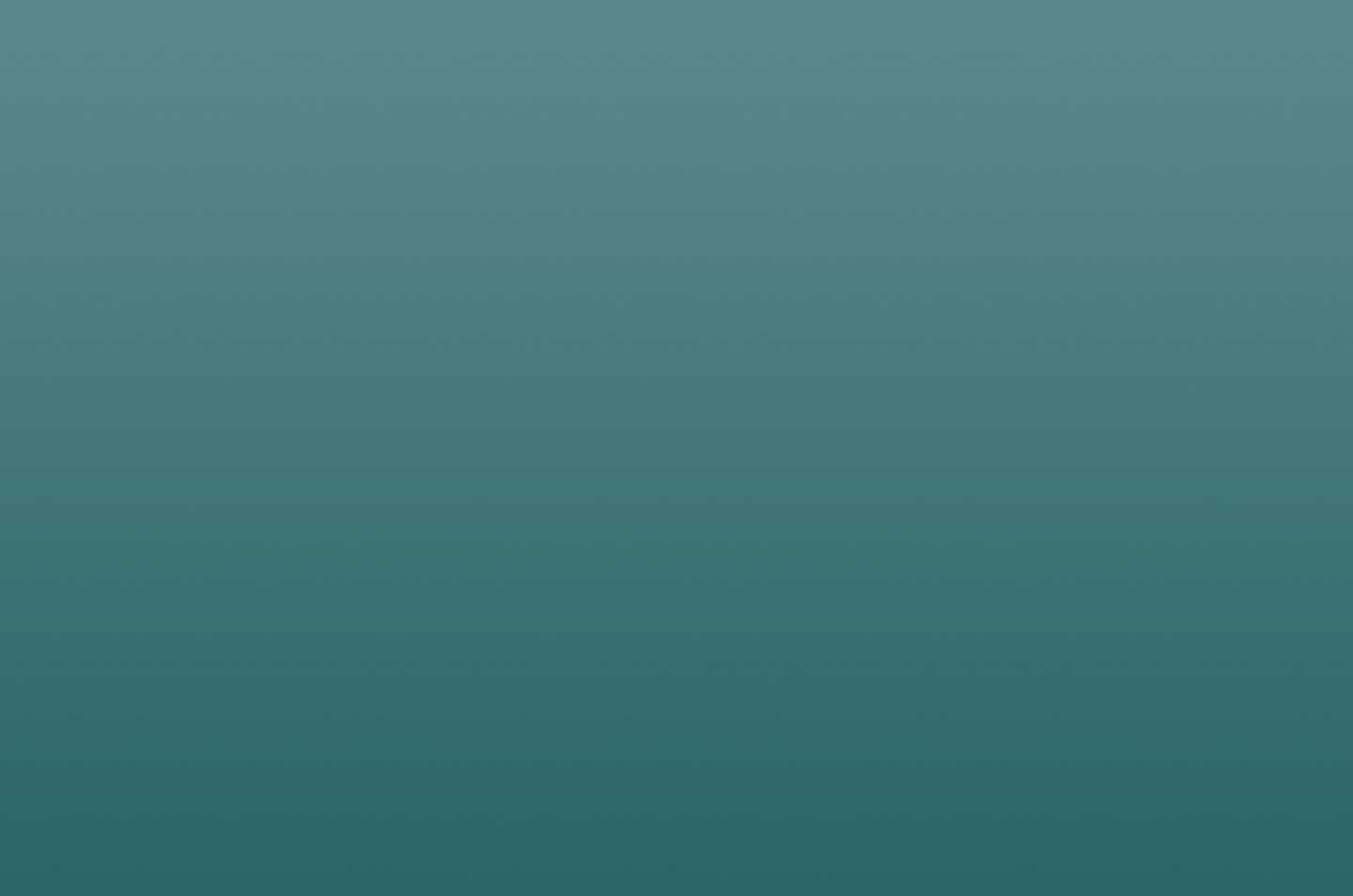
As hardware technologies advance at a rapid pace, it’s becoming possible to build powerful AI applications that deliver valuable insights in real time. The key is implementing inferencing at the edge to reduce latency and accelerate time-to-insights. By leveraging the latest hardware, technology developers are enabling a new era of hyperautomation with edge computing.
Read on to learn how edge computing enables ultra-low latency inferencing, and in turn, real-time AI use cases. We’ll also discuss how to accelerate the development of edge devices for real-time AI applications using pre-designed hardware.
The Need for Low Latency AI Processing
In the field of machine learning, latency is the time it takes to generate insights from new data collected, which has always been a technological challenge for developers. If an AI application takes too long to generate insights, its value is limited to ad hoc use cases rather than driving decision-making in real time. That’s why optimizing latency, throughput, and other performance factors is always a priority for resource-intensive AI and machine learning applications.
While there are many factors that impact latency — such as the efficiency of the machine learning model and even the software itself — the network latency of cloud-based applications can cause significant delays. In fact, high latency and throughput constraints have prevented many use cases that require on-demand insights in the past.
However, optimized machine learning models, modern application architectures, cutting-edge hardware, and other technological advances are enabling low latency AI processing. This is driving real-time use cases, including:
- Medical: AI-guided surgical system robotics can aid not only executing tiny, precise movements, but also with providing real-time information about the condition of a patient. These insights, which are only made possible with low latency AI and computer vision, help clinical professionals with surgical tasks to ensure the safest and most precise results.
- Physical Security: AI can be integrated into traffic and physical security systems to improve the safety and security of public areas. This requires real-time insights that can detect people and vehicles using computer vision to help human personnel deal with security incidents promptly.
How Edge Inferencing Reduces Latency
Although many of today’s AI applications currently rely on cloud-based inferencing, this approach has its drawbacks. Transferring data to the cloud for processing introduces latency depending on network conditions and the availability of high-speed Internet connectivity. In addition, bandwidth constraints can slow the uploading of large datasets and the downloading of results.
Edge inferencing reduces network latency because processing occurs on the embedded system or on a nearby edge server within the local intranet. In general, the closer inferencing is implemented to the data source, the faster insights can be generated by AI applications. Even insights from massive life sciences datasets or analytics for high-resolution video streams can be delivered efficiently using edge computing.
Further Reading: Medical AI: From Cloud to Edge Inferencing
Besides reducing network latency, edge inferencing can leverage increasingly powerful hardware to further accelerate machine learning applications. For example, leading hardware manufacturers are developing solutions like NVIDIA Jetson, Google Coral, and Hailo that provide high-efficiency, small form-factor embedded computing boards and acceleration modules designed to run at the edge.
Developing Edge Devices for Real-Time AI Applications
Edge devices were previously out of reach for many technology developers, but they’re now more economical and practical than ever before. Hardware has become cheaper and faster, with smaller footprints and lower energy demands. These technological improvements have sparked a new wave of edge AI solutions in manufacturing, healthcare, and many other industries.
As the total cost of ownership for edge-based inferencing continues to fall, and the demand for real-time analytics and hyperautomation increases, technology developers will need to adapt their solutions to meet these new expectations. A trusted hardware partner like AHEAD can offer strategic guidance for designing, building, and integrating a high-performance edge solution.
AHEAD is a hardware specialist with comprehensive services for streamlining the development of AI edge computing solutions. Our building blocks approach combines pre-designed hardware with customization to meet specific use case requirements. This greatly reduces the cost and time-to-market for embedded systems, edge devices, and edge servers for real-time AI applications.
Get in touch with us today to learn more.