OVERCOMING DATA CHALLENGES RELATED TO AI
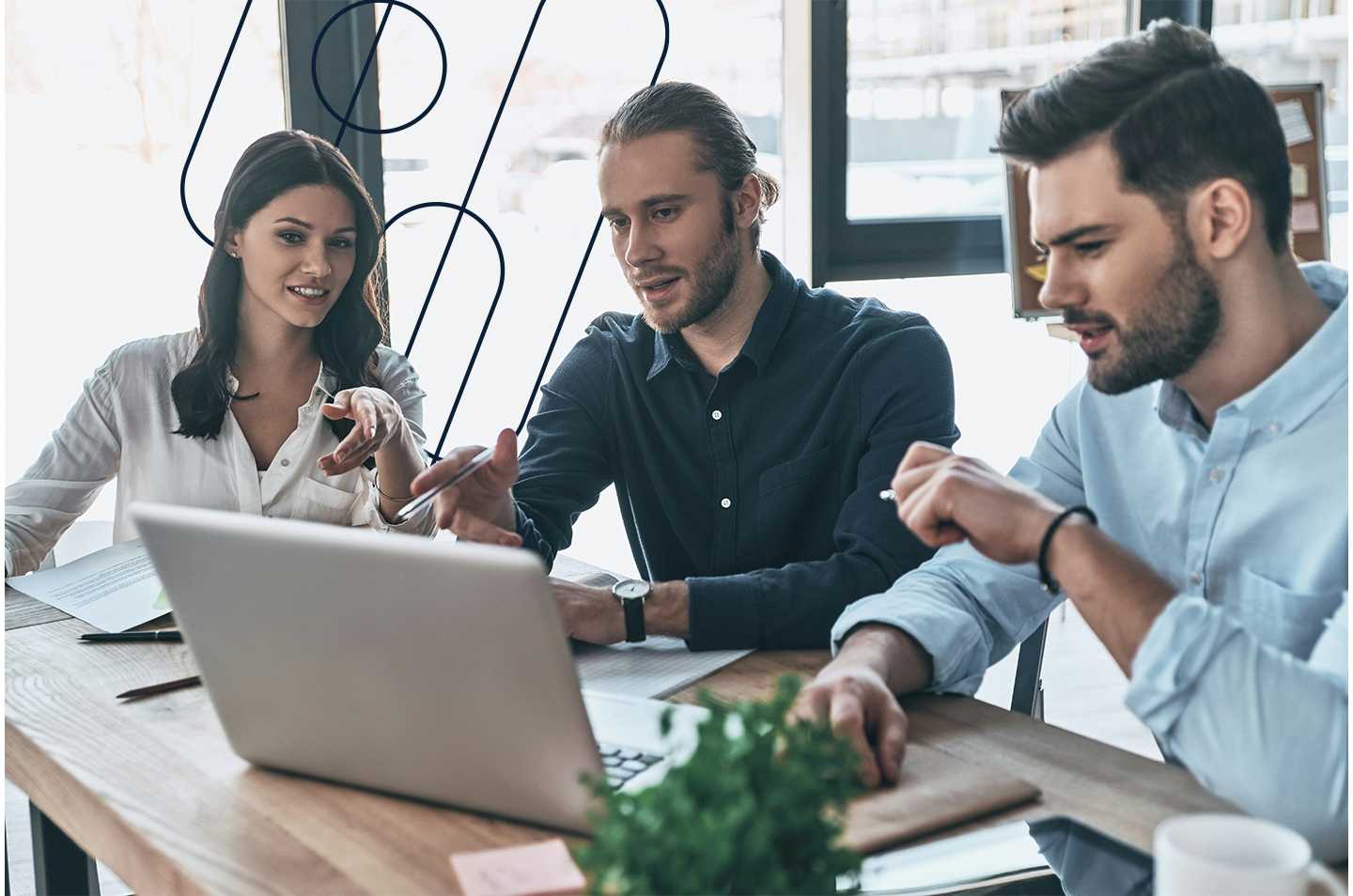
It should come as no secret that immense value exists in the business application of artificial intelligence. We see new stories come out every day on what AI is capable of and what it’s doing for organizations. That said, most companies aren’t yet leveraging AI to its full potential – or at all. And while there is so much upside to utilizing AI, there are also several challenges – many specifically related to data – that are holding them back. Below, we’ll explore these challenges and offer guidance on best practices for overcoming them.
Data Quality
It is all too common for organizations to experience issues with data quality or a lack of trust in their data. This can stem from various issues, such as incomplete or inaccurate data collection processes and lack of data validation or quality assurance processes.
These data shortcomings can lead to inaccurate insights and fractured decision-making processes, which stifles the ability to leverage AI effectively. If an organization cannot trust their data and the insights driven by it, they won’t be able to trust the AI applications being fed that data.
- Solution: Establish data quality standards with clearly defined guidelines and objectives, including benchmarks for accuracy, completeness, consistency, and reliability.
Data Integration
Large organizations, who otherwise have more resources to allocate to AI initiatives and fewer roadblocks to avoid, frequently struggle with data integration as it applies to artificial intelligence. This is often due to data silos across different business units, departments, systems, and applications. While organizations have spent years focused on breaking down and preventing data silos, there are several tough-to-avoid root causes for silos. For example, data residency laws (e.g., GDPR, CCPA, PIPEDA) that vary by location and/or contain heterogeneous data formats (e.g., structured, semi-structured, unstructured) can be difficult to join together.
No matter the cause of these data silos, they tend to lead to incomplete or narrow-minded insights, which are not conducive to effective or trustworthy AI systems. When individual pockets of data exist, organizations cannot assess the full breadth of their data and instead must rely on a view of just one area or system at a time. This can lead to manual and fragile integration processes that ultimately create more work for data teams.
- Solution: Define an architecture, such as a central modern data platform, that is capable of handling various data types and has tooling for ingestion from a number of systems. These systems can include major database management systems (DBMS), cloud storage solutions, and SaaS and PaaS offerings.
Data Security & Privacy
Organizations today have more concerns than ever before – from data breaches and cybersecurity threats to ransomware and data accidentally being made public. And while security is top of mind for most enterprises, many do not realize that they are falling short, leaving them unable to validate the security of their data. Insecure data may lead to loss of customer trust, regulatory penalties, legal implications, and damage to reputation, all of which can be extremely difficult to recover from.
These data security concerns are even more pronounced when it comes to artificial intelligence, as organizations must ensure that the data being fed to AI systems is monitored and validated each step of the way. Failing to do so can greatly increase the likelihood of running into the issues mentioned above.
- Solution: Conduct comprehensive risk assessments to get a baseline for security vulnerabilities across the organization. This will help ensure that security teams are aware of potential risks and allow them to proactively resolve issues. Organizations should also implement robust security measures that include multi-layered controls, such as encryption, firewalls, and automatic security updates. Finally, be sure to train ALL teams on security standards/practices and consider implementing policies such as ‘zero trust’ or ‘least privilege access.’ Each of these steps will help to ensure that your data is secure, and in turn, safe to use in the development of AI solutions.
Data Governance
Yet another impediment to successful AI initiatives is the lack of a clear data governance framework. While most organizations understand the importance of data governance, many fail to establish a true culture of data governance, which can result in inadequate funding and awareness, data inconsistencies, and poor data quality. Further, a lack of governance stifles data management, limiting the ability to implement policies over the data. All of this compounds over time, making it difficult for leadership to make informed decisions for the future of the organization.
With regard to AI, poorly governed data leads to poorly trained systems, and thus, untrustworthy results. For example, if metrics are being calculated differently throughout the organization, the logic behind those metrics will vary greatly. And because AI systems leverage this data, the inconsistencies will hamper the ability of the system to provide the desired outputs.
- Solution: The overall goal should be to create a clearly documented semantic layer that all data and AI use cases leverage. To achieve this, establish a data governance framework by defining governance policies and procedures, assign data stewards throughout the organization, implement data governance tooling and technologies, and foster a data-driven culture across the enterprise.
Data Analytics & Insights
Too often, organizations fail to establish teams capable of building analytical applications, and therefore struggle to effectively analyze and interpret data to make informed decisions. Without effective data analytics, the organization may miss valuable business opportunities (sometimes, without even knowing it). If decisions made throughout the organization are led by incomplete or inaccurate analytics, the results will almost certainly be misaligned with business goals, which will ultimately render AI applications ineffective.
- Solution: Define clear objectives, identify all relevant data sources, and utilize industry-standard advanced analytics techniques. Investing in data analytics tooling and technologies will serve as a vital first step to building a data analytics center of excellence, enhancing the organization’s ability to make decisions while paving the way for AI use case and application development.
Data Ownership & Collaboration
When it comes to data ownership and collaboration, organizations frequently struggle to clearly define who is responsible for the management, maintenance, and security of their data. We often see situations where one team owns the application producing the data, but another team is tasked with storing it, and yet another with securing it. This disjointed data management leads to a lack of trust and accountability when issues arise. This particular challenge of clear data ownership and management only heightens each of the aforementioned challenges, and ultimately creates an environment where AI applications are that much harder to develop effectively – or at all.
- Solution: Establish clear data ownership and communicate roles and responsibilities throughout the organization. By creating data governance committees with cross-functional teams for resolving data ownership conflicts and implementing seamless data sharing via modern data platform tooling, organizations can ensure that consistent, high-quality data is being used to feed AI systems.
Final Thoughts
Before making significant investments in AI, it’s critical to address the challenges listed above. Doing so will not only accelerate your AI journey but improve the decision-making process throughout the organization. These challenges don’t align with any single function or department, but instead, are a cornerstone of data management fundamentals that apply to everyone. Keep in mind that data challenges cannot be solved with a one-time fix or investment – they require continuous attention that needs to be supported from the top down to become truly data-driven, which is a precursor to building high-performing AI applications.
To learn more, get in touch with AHEAD today.
About the author
Ross Stuart
Principal Technical Consultant
Ross Stuart is a Principal Enterprise Architect with 10+ years of experience with Big Data, Machine Learning, and Cloud Computing. Ross has engaged with numerous Fortune 100 companies on data migrations, cloud transformations, and everything in between. His extensive background in cloud computing and solving data problems through modern data platforms has helped provide valuable solutions to numerous AHEAD clients.
RECOMMENDED RESOURCES
Adopting AI Lifecycle Governance to Deliver Reliable, Transparent, and High-Performance AI Systems
Read Article

Charting a Path from Data Science to Large Language Models & AI
Read Article

Unlocking AI’s Potential: Challenges and Opportunities for Data Leaders
Read Article
