OPERATIONALIZING AI AT THE EDGE IS THE NEXT AI BATTLEGROUND
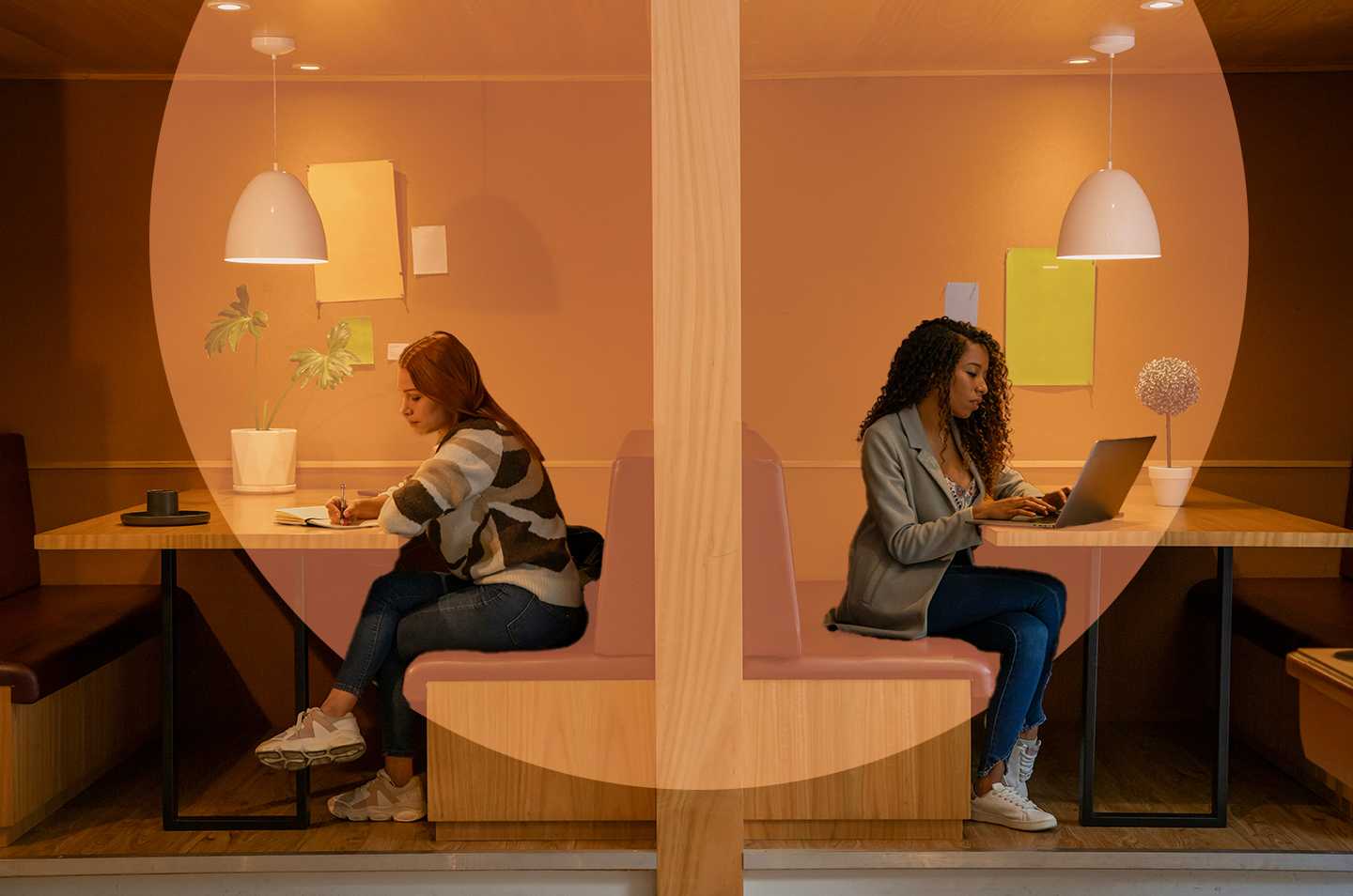
This article was first published in VentureBeat.
The next AI battleground
As more organizations charge into the AI and machine learning fray, technology and operations leaders are keeping one eye on their competition, and the other on how their own AI workloads are impacting their infrastructure needs now — plus worrying what the near future will demand in terms of evolving technology and sophisticated infrastructure.
There’s plenty to worry about. The number, size and complexity of AI workloads is skyrocketing, which is alarming news for data centers, which are already pressed to the limit trying to power, cool and find space for the high-octane infrastructure required to stay just ahead of the ravening horde. On the chipset side, innovation is a blur, giving companies behind large foundational and frontier models the power they need to keep pushing those models forward, and making them ever larger and more computationally complex.
The hardware that started the AI revolution is arguably antiquated now for the current model sets, and refresh iterations are getting shorter all the time. Where an organization could expect to keep infrastructure for five to seven years, they’re finding that number shrunk to months-long lifecycles instead. Space issues magnify, as companies scramble to make room for migrations and growth.
There are some clear directional signals, says Mike Menke, Field CTO at AHEAD, and key among them is the push towards edge computing across industries.
“We’re talking about very power-hungry, very large systems,” Menke says. “While we’re going to alleviate some of the data center constraints, we’ll continue to push the boundaries of what we can do at the edge and the far edge as we try to get the computational part of it closer to the end consumer or user. The edge is where inference is ultimately going to end up.”
Edge adoption across industries
Edge computing is used widely in a broad array of asset-intensive industries, like manufacturing, utilities, retail, healthcare and transportation and logistics, where companies are pairing live streams of data from an ecosystem of edge sensors and IoT devices with AI to make real-time decisions.
Adoption is growing, too — Gartner predicts that by 2025, 75% of enterprise-generated data will be created and processed outside a traditional centralized data center or the cloud, and a big chunk of that growth comes with new use cases across all industries.
A growing demand for machine learning, AI and low-latency data analytics will continue to accelerate the demand for edge computing solutions. Edge AI can collect and analyze data and deliver insights that drive efficiency, reduce safety and security risks, and help organizations deliver more value to customers. It’s also applied in use cases that require lower latency and data gravity (or the need to reduce bandwidth costs by processing data where it’s generated), and where greater resilience is needed during disconnection.
That includes not just physical security systems using computer vision to detect and report safety and security incidents, but data-driven clinical decision support (CDS) solutions for healthcare providers. And as the demand for real-time analytics continues to grow, organizations will require more data processing and inferencing at the edge, and ongoing rapid innovation in distributed cloud solutions, device orchestration and management software, edge application platforms and other technologies will keep driving edge adoption.
Edge and far edge, now feasible at scale
Implementing edge solutions has been a challenge until now, for a variety of reasons says Bill Conrades, Senior Director of Engineering at AHEAD.
“Edge AI isn’t just the hardware and the software, it’s also the sensors involved in generating the live stream of data,” Conrades says. “The vendor ecosystem is extremely fragmented and most IT departments aren’t set up to integrate and maintain their edge devices at scale. You need supply chain transparency, asset management, integration and configuration services, plus life-cycle management transparency, so you know when to refresh your licenses and support contracts.”
And as AI and generative AI use cases multiply, organizations have run up into all-too-common roadblocks, including the need for major engineering, data science and IT infrastructure investments that require a serious number of tools and a whole lot of expertise that’s fairly thin on the ground these days, no matter the industry.
Some significant factors have changed the game, making far edge, in particular, more feasible. Edge management and orchestration platforms (EMO) allow zero-touch provisioning and upgrades in the field, which is a huge deal in industries that work with far-flung and difficult-to-access locations, like oil rigs, solar farms and rail lines that span the country.
Most of those deployments have hundreds, if not thousands, of edge devices inferencing out at the far edge, and far from the core data center where the training takes place — and where the IT staff lives. With an (EMO) solution, the IT team can monitor sensors and the status of the system, deploy updates to the core AI model itself after it’s been fine-tuned with additional new data sets or parameters and update the hardware’s firmware if necessary.
“A lot of the customers see the benefit in managing their edge applications just like they manage their cloud applications,” Conrades says. “They want to manage their systems in the same way. A lot of these edge orchestration solutions support containers or virtual machines. They also support passing through the accelerator to the application with very little latency.”
Getting ready for the future
Organizations need to prepare to meet both the current state of AI as well as its future demands, and they’re both endlessly moving targets.
“A lot of organizations are used to having finite timelines on investments, projects taking a certain time,” Menke says. “Today it’s understanding that this is early days, and innovation is happening very quickly. You may be working on a problem and you wake up tomorrow and somebody on the other side of the world solved it while you were asleep. Being willing to grab that, bring that into your development process and take advantage of it is more important now than it’s ever been.”
Conrades points out that AI isn’t new at all — generative AI has simply brought traditional AI, including machine learning and deep learning more clearly into the spotlight, prompting more organizations to discover exciting new use cases. That means the wellspring of professional expertise available is deep.
“For instance, pull in a long-standing ISV that has a developed solution for your use case, operationalize AI and start solving today. Partner with an organization like AHEAD that can bring resources to the table to help you in your AI journey with pre-trained models, fine-tuning expertise, building blocks of hardware and a program that makes it easy and far faster,” he says. “I guarantee your competition is already picking up and operationalizing AI in some way shape or form.”
Learn more about AHEAD AI solutions and our edge capabilities.