NVIDIA GTC 2025 PERSPECTIVE: FIRST YOU GET THE POWER, THEN YOU GET THE MONEY
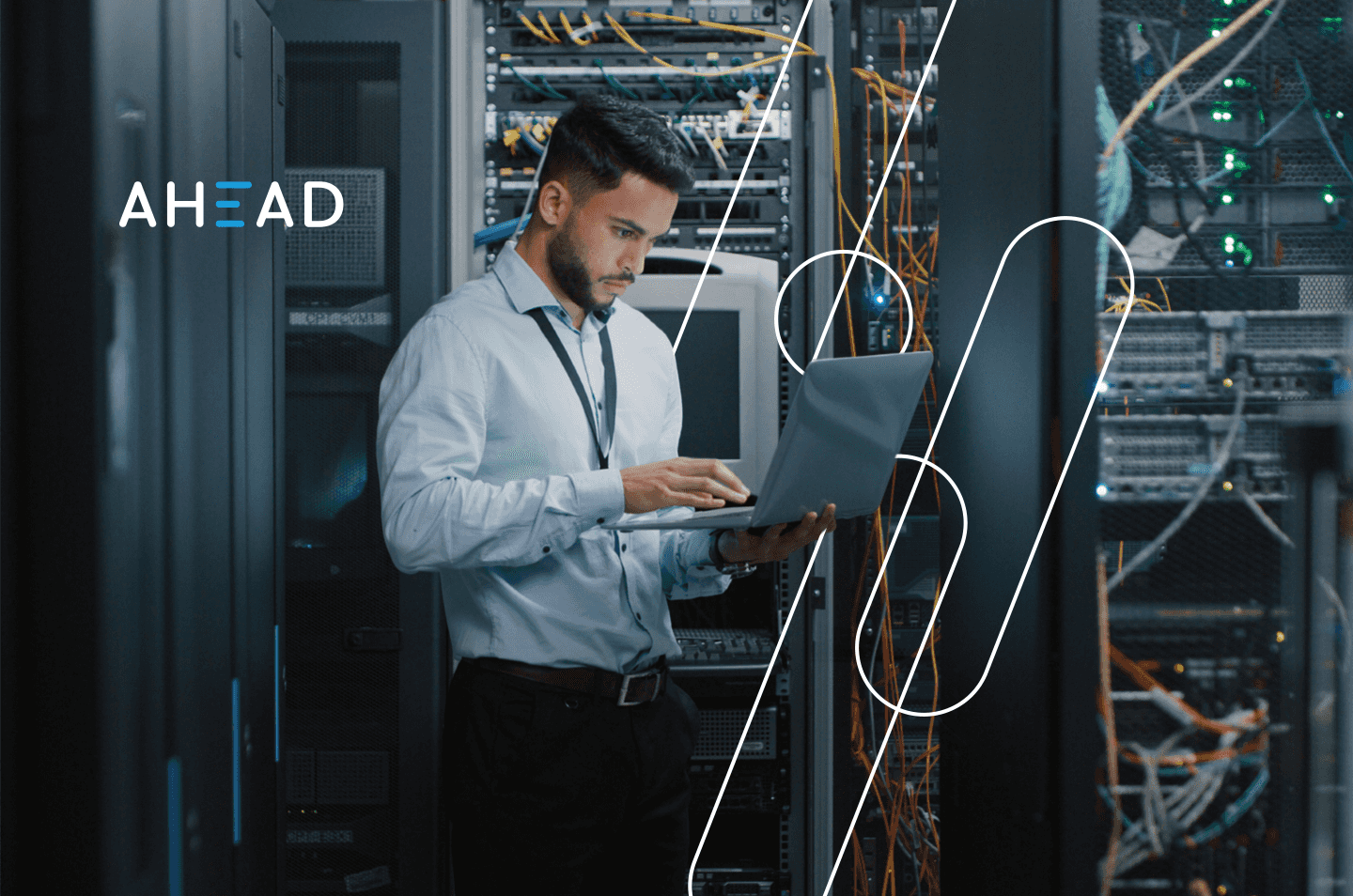
“…first you get the money, then you get the power…” is the original line delivered by Al Pacino in the 1983 film Scarface, and he almost perfectly summarized the main theme of NVIDIA GTC 2025.
We just need to flip it around to “first you get the power, then you get the money,” and we have the main hypothesis of this year’s conference: the successful enterprises of the future will be the ones who secure access to data center power today and most efficiently convert that electric power (via GenAI applications) into revenue-generating tokens as soon as possible.
Let’s explore the three key takeaways from GTC that support this hypothesis:
1. Power is scarce and becoming ever more so
2. Model complexity drives infrastructure homogeneity
3. Speed to AI infrastructure consumption is your primary success vector
1. Power is Scarce
This isn’t necessarily a ground-breaking observation, but it’s interesting to consider why power is a challenge today, and what will exacerbate the problem over time.
If you sat through any infrastructure-focused sessions at GTC, you probably heard about the rise of purpose-built AI data centers in response to how hard it is to upgrade existing data center electrical infrastructure. There are issues with permitting, local power regulations, and grid supply bottlenecks.
With IDC predicting that AI workloads are going to drive power demand to double from 397 in Terawatt Hours (TWh) in 2024 to 915 TWh by 2028, it makes sense for enterprises to turn to dedicated AI-hosting facilities to run their AI workloads. But, with nearly 84% of enterprises viewing AI as the next enterprise-critical application stack, there’s going to be unprecedented competition for access to these AI data centers. Further, inference workloads driven by AI reasoning models are 100x more compute-intensive because of the internal token generation/consumption required during complex thinking, reading, and reasoning tasks.
To make matters more interesting, the usable economic life of a GPU is shortening to roughly match the NVIDIA hardware development cycle. We have GPUs being released every 12-18 months that, in the case of Blackwell™ versus Hopper™, provide a 25X-40X gain in token generation at iso-power. NVIDIA is effectively financially obsoleting all prior GPU generations with each successive GPU release.
At this point the astute reader would ask me, “if I’m generating 40X more tokens at the same power draw, how does that lead to increased competition for power access?”
Great question!
Generating more tokens/kW represents a resource efficiency gain – and when resource consumption becomes more efficient, we tend to see more consumption of that resource in aggregate. The phenomenon is summed up as Jevons Paradox, named after economist William Stanley Jevons, who observed that efficiency in resource use can paradoxically lead to increased consumption of that resource, rather than decreased consumption, due to factors like lower entry-point costs and expanded availability. For example, when gas prices drop, people tend to drive their cars more frequently.
Finally, the 3-year roadmap for AI factory power consumption is projected to be an order of magnitude greater than what most companies have deployed today (A100/H100/H200). As stated in the keynote, the GB300 NVL72 is projected to draw ~140 kW per rack. In late 2027, the Vera Rubin Ultra NVL576 is expected to draw 600kW per rack.
So, the message regarding power was loud-and-clear: Reserve your seat now or you might get left behind.
2. Model Complexity Drives Infrastructure Homogeneity
Assuming you’ve procured the requisite power capacity, what do you do now?
Maybe you have dozens or potentially hundreds of product teams, researchers, labs, principal investigators, data scientists, and developers with a range of AI infrastructure and software needs. Each may have a unique budget, a grant, an opinion on hardware, a preferred software stack, and unpredictable demand for training versus inference compute.
Add in the fact that each persona might configure any number of different models with varying configurations for data parallel, pipeline parallelism, tensor parallelism, expert parallelism, inflight batching, and kv cache management, and you have an impossible nightmare-matrix of requirements to meet.
So, again, what do you do now?
At scales like these, AHEAD has found success helping our clients build shared and centralized clusters for training and experimentation workloads. When we homogenize the hardware and leverage an opinionated stack of management software, clients are able to accelerate cluster adoption and maximize cluster utilization.
What this means in practice is adopting a prescriptive approach to AI infrastructure deployments and programmable logical configurations.
We can accomplish our goal of rapid and repeatable builds by adhering to Reference Deployment Guides (RDGs), OEM Validated Designs, and Reference Architectures (RAs), like NVIDIA® DGX SuperPOD™ and BasePOD™, which provide concise design guides for implementing AI hardware at scale.
But, like any reference architecture, NVIDIA’s has some flexibility, and working with a DGX SuperPOD-Certified partner like AHEAD can help you get the most out of any customizations and integrations that need to be made.
Of course, we can also swap any layer of the hardware (compute, network, storage) with your preferred OEM to meet certain design goals around power, cooling technology, CPU chipset, memory ratios, storage tiers, etc. that may fall outside of the official DGX SuperPOD spec, but are nonetheless technically valid.
When workloads don’t fit into a centralized deployment pattern, AHEAD can custom-design edge AI inference appliances for even the most demanding environments.
But still, how could one AI infrastructure serve the majority of researchers and data scientists we described above? The trick is in the NVIDIA Dynamo™ and Mission Control™ software stacks that allow homogenous AI infrastructure to be dynamically programmed for changing concurrent AI workloads.
As an example, perhaps model training and experimentation dominate the AI infrastructure in the mornings, while in the evenings, inference demand skyrockets. Instead of building two pools of infrastructure, we can use the NVIDIA Dynamo disaggregated inference server software and NVIDIA Mission Control to make a single DGX SuperPOD behave as a dynamic pool of training/inference and dev/prod, compute capacity with built-in horizontal scaling, workload restart, and health check services.
The main takeaway: NVIDIA’s AI DGX SuperPOD reference architecture is being used in every vertical to serve an evolving set of workloads, and NVIDIA Mission Control and Dynamo software are going to be key enablers for making AI infrastructure dynamic and programmable.
3. Speed to AI Infrastructure Consumption is Your Primary Success Vector
Now, let’s assume we not only have requisite power, but also a solid AI infrastructure and software proposal design in-hand. They want us to spend $30M on all this stuff, and it’s going to take months to implement! We just learned that rapid NVIDIA GPU advancements mean the useable economic life of GPUs is 18-24 months. Maybe we should do nothing and wait for the next-generation GPU to come out so we can produce more tokens, deliver drug experimentation, diagnostic techniques, simulation outcomes, etc. 40x faster at iso-power?
No! You’ll be stuck in a cycle of always waiting for the next widget.
The solution isn’t to wait and see; the solution is to thoroughly vet capable AI partners who will help you minimize the time to full-go operation so you can realize the most return on your AI infrastructure investment.
AHEAD has extensive experience designing, integrating, deploying, and managing custom large-scale AI/HPC infrastructures, NVIDIA DGX SuperPOD, NVIDIA BasePOD, OEM solutions (think HGX from Dell, SuperMicro), and hybrid/cloud infrastructures.
In this domain, AHEAD has helped clients compress deployment timelines from months to weeks, and weeks to days.
How does AHEAD accelerate these timelines? To understand, let’s explore why a do-it-yourself AI factory deployment could take months:
- You spent weeks reconciling shipped versus received inventory
- You spent weeks unboxing 3,000+ components like DAC cables, transceivers, fiber, GPU nodes, switching, power/rack components, and the like
- Someone didn’t plan power distribution and corresponding layout for 30 racks correctly
- Cabling took a few weeks
- Power cords are wrong
- Fiber is the wrong length
- Cluster tests confirm several cables, transceivers, and nodes are degraded and need replacement
- You wait several more weeks for replacement components to show up because component failure at scale wasn’t accounted for in the initial order
- Re-testing takes a few weeks
Now, with an operational cluster, you still have to triage which researchers and product teams get onboarded first. After all, a powered-on cluster does nothing until your teams use it, but perhaps your infrastructure teams don’t have the hours in the day to do things like set up the network connections and file transfer portals that enable self-service migration of data from around the enterprise. Maybe they don’t have time in the day to rewrite user-facing cluster documentation; maybe they don’t have time to evangelize the new AI infrastructure and perform user outreach; maybe they don’t have time to run educational user-onboarding workshops that demonstrate the art of the possible to drive cluster demand.
All these reasons and more are why it is critically important to partner with a team like AHEAD that understands AI planning, AI infrastructure, and AI operations at scale.
At AHEAD, our FoundryTM manufacturing and integration facility accelerates complex AI infrastructure deployment timelines by up to 85% by conducting end-to-end infrastructure integration, testing, and pre-deployment. Pre-configured rack-scale AI solutions leave AHEAD facilities in a consistent foundational state after node/OS installation, system configuration, health and DOA checks, AI/HPC benchmark and burn-in testing, and workload management and orchestration testing.
Totally custom AI/HPC infrastructure? We do that. DGX SuperPOD and BasePOD from NVIDIA? We do that. HGX liquid-cooled from SuperMicro, Dell, etc.? We do that. Kubernetes cluster with Run:ai workload orchestration for the GPU nodes, and a standard slurm setup for the HPC nodes? We do that. Data center rack-scale cabling and layout design, including component labeling? We do that.
And at AHEAD, we don’t stop at simply building and deploying the digital shovel; we’ll dig alongside our clients’ teams to ensure we are driving utilization and adoption through a combination of next-generation managed services offerings.
We pair traditional 24x7x365 infrastructure managed services with agile-based Accelerate Teams to ensure the fastest possible cluster adoption in client environments. Infrastructure break-fix, change management, NVAIE/HPC software configuration, user-onboarding, data migration, AI use case intake, and extension to cloud environments are among the things our teams are asked to do on a daily basis.
Final Thoughts
If you want to go far and you want to go fast, choose to partner with a team that’s done it before.
In recognition of AHEAD’s technical leadership in the design and deployment of NVIDIA software, NVIDIA DGX™ systems, NVIDIA HGX™, and networking technologies that advance AI, AHEAD has been named the 2025 NVIDIA® Rising Star Partner of the Year.
We’re looking forward to seeing what our existing clients do with this technology, and how we can partner with you to build something great!
Get in touch with us today to learn more.
About the author
Vinnie Lee
Client Solutions Engineer
Vinnie Lee is a Client Solutions Engineer serving AHEAD’s Northeastern clients. He has spent the last 5 years at AHEAD helping clients build cloud environments, design digital platforms, and implement enterprise service delivery frameworks by leveraging best-in-breed technology and design.