MOVING BEYOND AI PARALYSIS
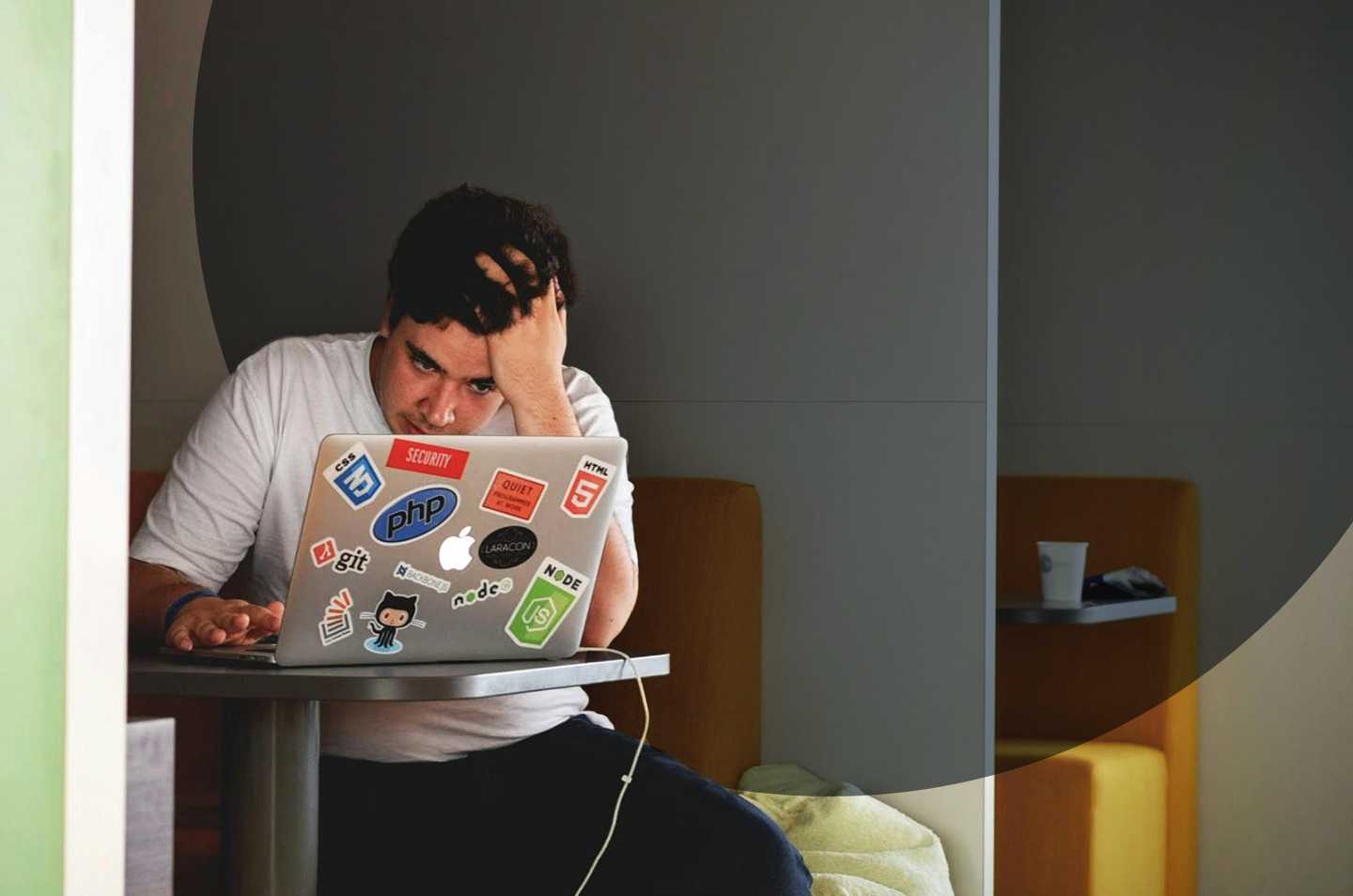
The global AI market has exploded, estimated at around $4 billion in 2014, to a staggering $200 billion today (July 2024). The number of AI startups continues to surge, up roughly 14X since 2000. People are leveraging AI in their everyday lives, as 77% of devices are anticipated to use some form of AI, even in washing machines. As capitalizing on this market growth is critical for people and businesses alike, the Saudi Arabian government just made a huge splash by starting a $40 billion dollar venture into the space and is currently looking for someone to lead the fund. The AI revolution is just beginning.
“Most organizations are gearing up for a headlong rush toward the adoption of generative AI to stay competitive, even as they’re feeling overwhelmed by the number of tools, paralyzed by choice between a myriad of potential use cases, and under pressure to deliver dramatic results,” says Ryan Barker, Field CTO at AHEAD.
The question remains: where to start? The slightly boring but very critical answer will always be with the data.
Having the Data Conversation
“Every AI conversation leads directly to a data conversation,” Barker says. “You need to align the right data to your AI use cases before you can get real value for your enterprise from AI.”
AI, of course, relies on data, and given that data volume is expanding at a wild speed, questions around quality, lineage and long-term storage are more critical than ever. Complicating the issue is the number of disparate tools and technologies used to access and manage that data, causing bottlenecks that hamper AI tools.
Assessing data readiness is one of the first areas AHEAD tackles for its clients,” Barker says. “And it kicks off with finding where the required data lives — there’s always a lot of it, and it’s frequently siloed and hiding in a huge number of places, whether on-prem, in the cloud, at the edge or even sitting on someone’s device. It could be forgotten in a legacy application and require unique methods of extraction, or safe but useless behind a variety of access controls.”
Even before tackling the data discovery process, it’s critical to ensure that a strong governance and data quality practice is in place as a foundation for best practices when cleaning the data. Luckily, there have been major advances in the tools and technologies that handle data governance and quality control. These tools can automate quality checks as the data is found and investigated since they’re able to understand the content and structure of information as it comes in.
“The data piece can end up being a pretty huge issue to tackle,” Barker adds. “Typically, we navigate clients through figuring out a data set to start with so it’s not overwhelming and you’re making concrete progress while you’re in the beginning learning stages. But it’s all about making sure we get the most value out of AI once we get to that stage.”
Defining Technology Investments
As one of Barker’s customers lamented, AI is the solution in search of a problem. Choosing a direction and the right tools to achieve success can be paralyzing. “Some companies are leaning into already-built tools like Microsoft Copilot to enhance their business processes right out of the gate, and that’s a good first step, but it doesn’t preclude other, larger investments,” Barker explains. Some of the advanced use cases will require enterprises to adopt more vertically aligned solutions and models to maximize the value proposition of those investments and get the most out of process change.
“When you make an investment with a platform integration, it’s typically specific for just that platform and not really shared across all applications and all data within an organization,” Barker says. “You’re limited. You’re locked in.”
Every organization struggles with knowledge management and deriving insights from disparate sources. For many, the first step is to build a scalable RAG framework that incorporates multiple data repositories to leverage across the organization.
Retrieval Augmented Generation (RAG) as a First Step
“We’re seeing a lot of companies get early value by leaning into building RAG architecture,” Barker says. “It’s probably the best place to make an investment, because it scaffolds all your AI initiatives by letting you extract accurate information, insights and value from your data — something that’s been notoriously difficult to do for decades.”
RAG architecture tackles the limited nature of LLMs, which are generally hemmed in by their training dataset. It can retrieve information from external sources, whether that’s publications outside the organization or proprietary company data within, to create a generative AI system that’s dynamic and relevant. Instead of needing to fine-tune and re-train an LLM every time there’s new information, RAG architecture adds necessary context to a user’s prompt, making it a far more cost-effective way to add specialized data to your LLM.
RAG adds a great deal of transparency, and the number of unchallenged hallucinations is dramatically reduced, because the LLM can point to the sources it used for its responses enabling users to verify the system’s claims. Answers are also improved by information that’s more up to date than the training dataset.
LLM-powered chatbots can leverage RAG to deliver more useful answers based on company knowledge bases, which improves the customer experience by making chatbot responses less generic and more relevant. Internally, generative AI-enabled search engines and knowledge bases are vastly improved with the addition of company data across an array of roles — for example, accounting can access financial databases, sales teams can query their CRMs and sales statistics and more, improving operations from the start, all done in natural language.
“I call it hybrid AI because you’re leveraging different pieces of technology, whether it’s in the cloud, on-prem, vector databases on top of your data or elsewhere, to build a platform that scales easily while you tackle more use cases over time,” Barker says. “It’s a great place for companies to start, and there’s so much value in creating an environment where users at every level of the organization can just ask questions about their own data and get quick answers.”
This article was first published in VentureBeat. You can find the original post here.