HOW AHEAD IS HARNESSING ADVANCED AI WITH THE HELP OF DELL TECHNOLOGIES
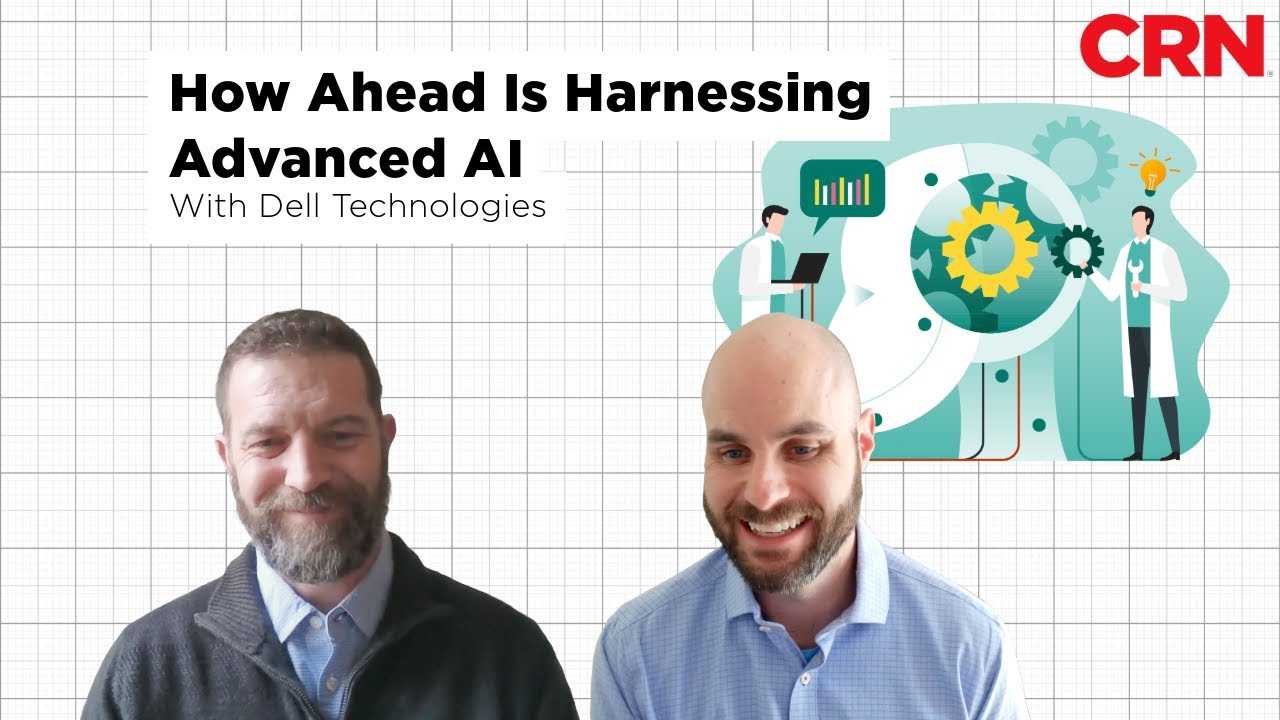
This video originally appeared in CRN.
Real World AI Applications with AHEAD and Dell
Chicago-based solution provider AHEAD has found success with real world applications that can harness the most advanced AI compute on the market to generate value for their customers.
AHEAD’s VP of Emerging Technologies Josh Perkins talked with CRN Senior Editor O’Ryan Johnson about the AI solution it created using a cluster of Dell Technologies XE 9680 servers to help a leading medical provider give patients a better outcome.
(A full transcript is below)
O’Ryan Johnson: I am O’Ryan Johnson, senior editor of data center and infrastructure at CRN, and I’m joined today with Josh Perkins, who is vice president of emerging technologies at Ahead. We’re talking today about some of the partnerships that are making AI profitable and actionable in the field.
Josh, just about any conversation you have in this space when it comes to AI eventually gets around to: What are you going to do with it? Where are you finding success? And at Ahead, you have found success in the real world with some use cases. Can you tell me a little bit about that?
Josh Perkins: Yeah, I think at the starting point we tend to want to focus very hard on the concept of applied AI, right? So not just generic use within organizations, but how are we actually disrupting something or reinventing or reimagining a process within the organizations we work with? And the one that I’m kind of most proud of recently is actually work in a nationally leading healthcare provider, Northwestern Medicine out of the Chicagoland area. Obviously, we’re exceptionally proud of being able to work with them, being that they’re right around the corner from us as an organization. But we have a very long-term relationship with them and they’re one of the leading hospitals in the country, so it’s an awesome circumstance.
OJ: Land of the Lincoln.
JP: Yeah, absolutely. And what we’ve kind of been working on with them and evolving over time and we were able to get connected with a brilliant clinician and researcher named Dr. Mazzi, who leads a team of both clinicians and technologists and also data scientists for the organization. And the goal of the group is to really be a kind of innovation engine for the use of technology and taking kind of the research side and creating applied work within the clinical setting for the organization that hopefully that, upon use, can then be kind of disseminated or distilled into kind of other organizations as best practices and built upon, right, for them. And the specific project around AI really is directly aligned to what they call the ARIES program. And ARIES stands for Automated Radiology Interpretation and Evaluation System and the concept here was that they wanted to be able to take a look at a tremendous amount of their image data and radiology information and start improving both the way that they detected, right? The consistency of the detection within scans to support their radiologists, integrate that natively within workflows because we didn’t want to disrupt clinicians through that process, generally speaking. And then also help them through the use of that tool to actually prioritize patient scenario. So, not just look at things and create productivity, but also try to get better outcomes from a patient perspective. And what’s really frankly just like, fantastically cool to me is they looked at this and said: “Okay, starting with something as simple as like a chest X-ray. How can we use a transformer architecture and generative models to be able to evaluate everything that’s going on in an X-ray and detect and understand and then develop actual human readable reporting that we can leverage out of that system?”
And they started by literally leveraging, I think it was 10 million images across all anatomies and an additional, I think 3 million-ish CT scans specifically of brain and chest functions with the goal of predominantly focusing on kind of key organ areas, right? Through this scanning technology. But the challenges that they ran into, like most organizations, is that in order to get access to resources, they really started by experimenting within their cloud environments. And we’re lucky enough, obviously, to have been able to help them build and leverage those environments as well. But over time, as their modeling efforts got more advanced and the data sets that they were working with got larger, it became really clear to that team that there was a bottleneck. They had a disconnect between the compute side of what they were working on and ultimately, the data side or the gravity that existed around these massive data sets that they would need to use in order to train and allow these models to continue to evolve and function within the organization. And so, it just became this pretty obvious structure even to non-technologists that we needed to fix that glitch, right? We needed to bring the compute closer to the information and create greater access across that team of brilliant researchers and technologists to get closer to the technology.
And hopefully the idea there was we could advance the efforts much, much faster or accelerate the progress they were making in that space. And I know your concept there was: “Well, what’s actual value, right?” Like it’s great if it’s research, it’s cool if it’s a tool that’s built in a lab, but what was the impact, right? What did they actually get out of using it? And the cool thing is that because they were actually integrating this directly into tools like PowerScribe 360, which is a native tool set for their radiologists, they were able to get a lot of radiologists using that right off the bat. In fact, over 40 radiologists across Northwestern now use this tool every day as part of their workflows. And the really key thing there is, okay, value creation beyond just the fact that it helped them better prioritize, frankly elevated the consistency of detect and presentation elements within those scans. But they also found after evaluating 11,000 co-scans with their radiologists that the radiologist saved on average 31 seconds per scan when they were evaluating, which is a 40.9 percent reduction in time per scan throughout the day. So, if we think about that, it’s not just like an efficiency or cost savings improvement in terms of the physician. It also allows those radiologists to look at more things throughout the day, see more patients, evaluate more ideas and scenarios for patients that ultimately lead to better outcomes downstream. So, it’s just been an amazing thing to watch and build with that team.
OJ: One of the critical components that you’re talking about, all the imaging that has to go on and the storage. I’m thinking about the storage and the infrastructure behind that. And you have to find a technology partner that’s going to be mature enough to be able to understand what you’re doing. And of course, with Northwestern medicine, that’s a whole other level of technology. As you make AI profitable in the real world, when it comes to partnerships, where are you looking when it comes to finding the technology partner who can do all those things?
JP: For sure. Well, I think first we’ve been really lucky to partner with highly innovative enterprise-class solution providers, traditionally speaking. And so, we’re really able to leverage a lot of our current platforms and relationships and just build upon and evolve with them as they continue to bring innovative and combinatory solutions to market. And Dell, by and large, is by far our largest single partnership within the organization. Deep roots, deep relationships, and frankly, just a strong belief that they deliver on what we ultimately need them to. And I think that that’s kind of a critical factor here is not only are they continuing to keep up with the pace of innovation and bring things to market, but they do that in a way that we believe they can support it, right? Which is really, really important because even though these researchers may be working on a science project, they don’t want the technology platforms that underpin those projects to be science projects too. They want them to be reliable and resilient architectures that blend into the operations that they already have with new organization and frankly get out of the way.
And I think partners like Dell and solutions providers like Dell really have just nailed that in terms of helping organizations continue to advance their cause without being massively disruptive to the way that they need to operate their technology.
OJ: Larger scale, looking beyond the Northwestern example here that you gave, what does a Dell partnership like this bring to the table when it comes to whether you’re talking to customers, whether you’re talking to prospects, does that sort of collaboration with somebody who’s as well-known as Dell, does that really matter?
JP: I think it matters quite a bit. To my point on de-risking, I think customers and enterprises have confidence in Dell platforms, right? They know that Dell has a history of not just showing up when they want to implement the technology with partners like Ahead, but also when we need to support things when things don’t go right. And I think that’s equally important in this scenario is when we’re doing really cool things and we’re pressing the edge of the capabilities of technology, it’s not always going to work the way that we intend. And so, it’s really, really critical that we partner with the right folks that understand that and build the support matrix around that to ensure that we can get back to where we need to be, both collectively as a partner and as a customer in that space. And then I think the other predominant concept there is just, I think there’s this opportunity whereby we’re making it easier for teams to adopt technology and wade into those investments appropriately. So, when we look at this and clients like Northwestern already had familiarity with a lot of the underpinnings of what ultimately needed to come together to build this new architecture environment for them. So, it wasn’t nearly the leap for their teams that maybe weren’t as involved in the tech to be able to consume those platforms and frankly integrate those into their environment in a way that lets them focus on the work at hand. And I think that the goal of AI is to create and innovate quickly, not just to deploy new hardware.