ENHANCING MLOPS WITH AZURE MACHINE LEARNING
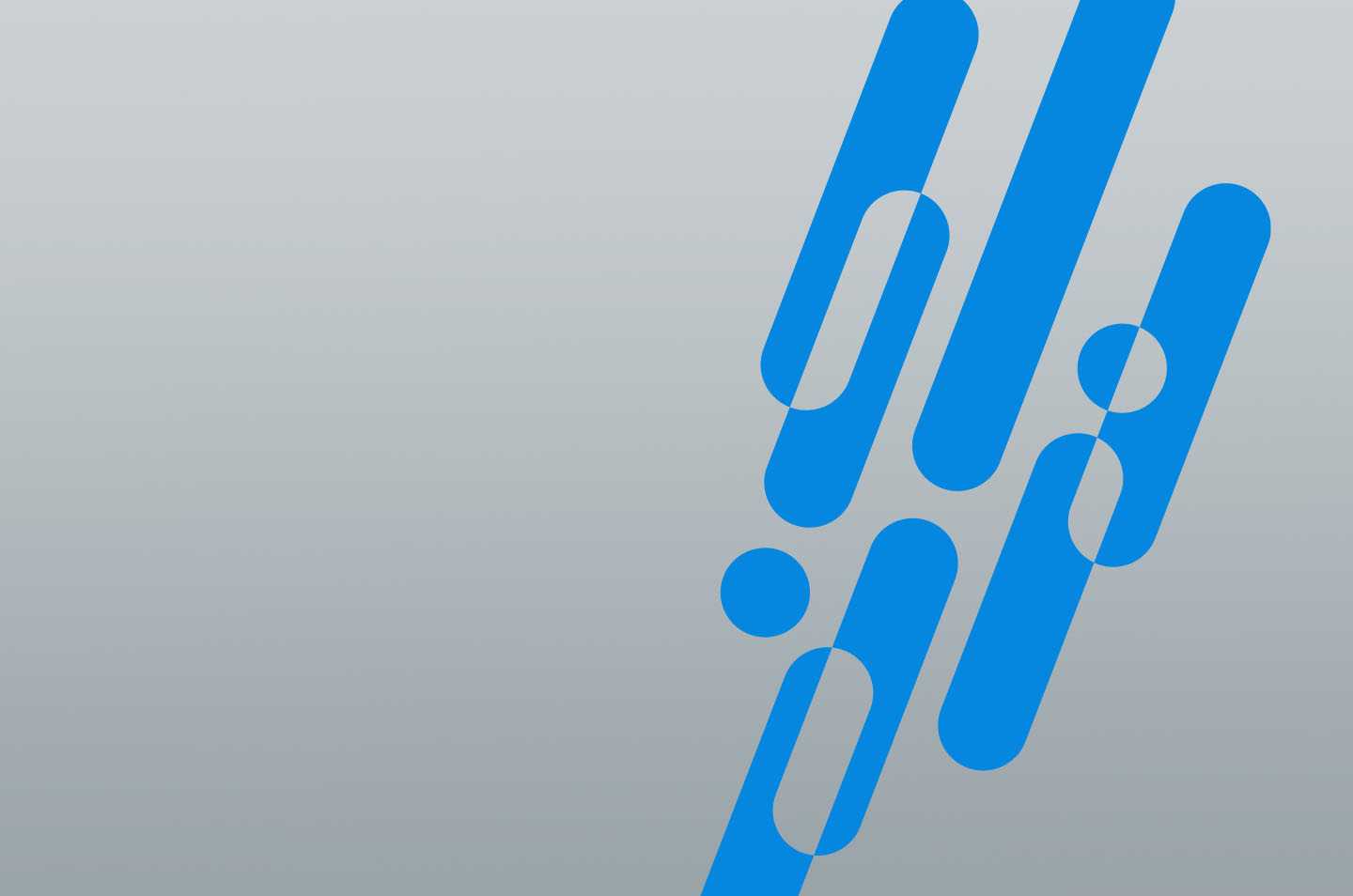
Recall the year 2013. The world has just topped four zettabytes of generated data (equal to four trillion gigabytes). The term “big data” is taking hold, creeping into every corner of the tech and business worlds.
From that point on, data volumes have grown exponentially. It’s estimated that the world produced more than 44 zettabytes of data by the end of 2020 (growing by a factor of 11 in just seven years). We’re now generating more data than we can effectively manage—and it’s a big problem.
Today’s organizations are searching for ways to harness, manage, and analyze these huge influxes of information. Many are turning to machine learning (ML) and deep learning (DL) models to analyze data and predict what could happen next. In fact, organizations across every industry use ML and DL today in various capacities.
Healthcare innovates pathology detection by leveraging neural networks and tracking patient patterns to predict their length of stay. The pharmaceuticals industry uses ML and DL to identify causal factors of diseases for drug development and predict patient response to drug combinations. Manufacturing organizations identify product defects, predict machine malfunctions before they even happen to provide proactive maintenance, and use generative design methods to determine the best structural design for a product (such as a car frame). From a general business perspective, many organizations leverage ML to aid in forecasting business revenue, schedule resources for projects, and drive sales and marketing efficiency.
But building and training effective machine learning models is no easy task. Add the maintenance (operations) component and achieving true machine learning operations (MLOps) is difficult for most organizations.
That’s why a platform like Azure Machine Learning can be beneficial. Azure ML is a hosted service that enables and enhances an organization’s MLOps capabilities through four key benefits:
- Graphical interface
- Out-of-the-box machine learning models
- Single pane of glass view
- Direct integration with Git and Azure DevOps
- Enables the use of Azure DevOps Pipelines to automate the data ingestion process and perform data checks
- Provides the ability to automate the Continuous Training and Continuous Deployment aspects of the MLOps lifecycle, removing the need to manually retrain models as performance degrades
- Automates scaling of compute resources for model training, both up and out, and provides a one-stop-shop for managing backend compute needs
- Automates deployment of trained/retrained models to internal- and external-facing services on Azure Kubernetes Services, Azure App Services, Azure Container Instances, or Azure Virtual Machines, and removes the need to manually deploy new or retrained models