CHARTING A PATH FROM DATA SCIENCE TO LARGE LANGUAGE MODELS & ARTIFICIAL INTELLIGENCE
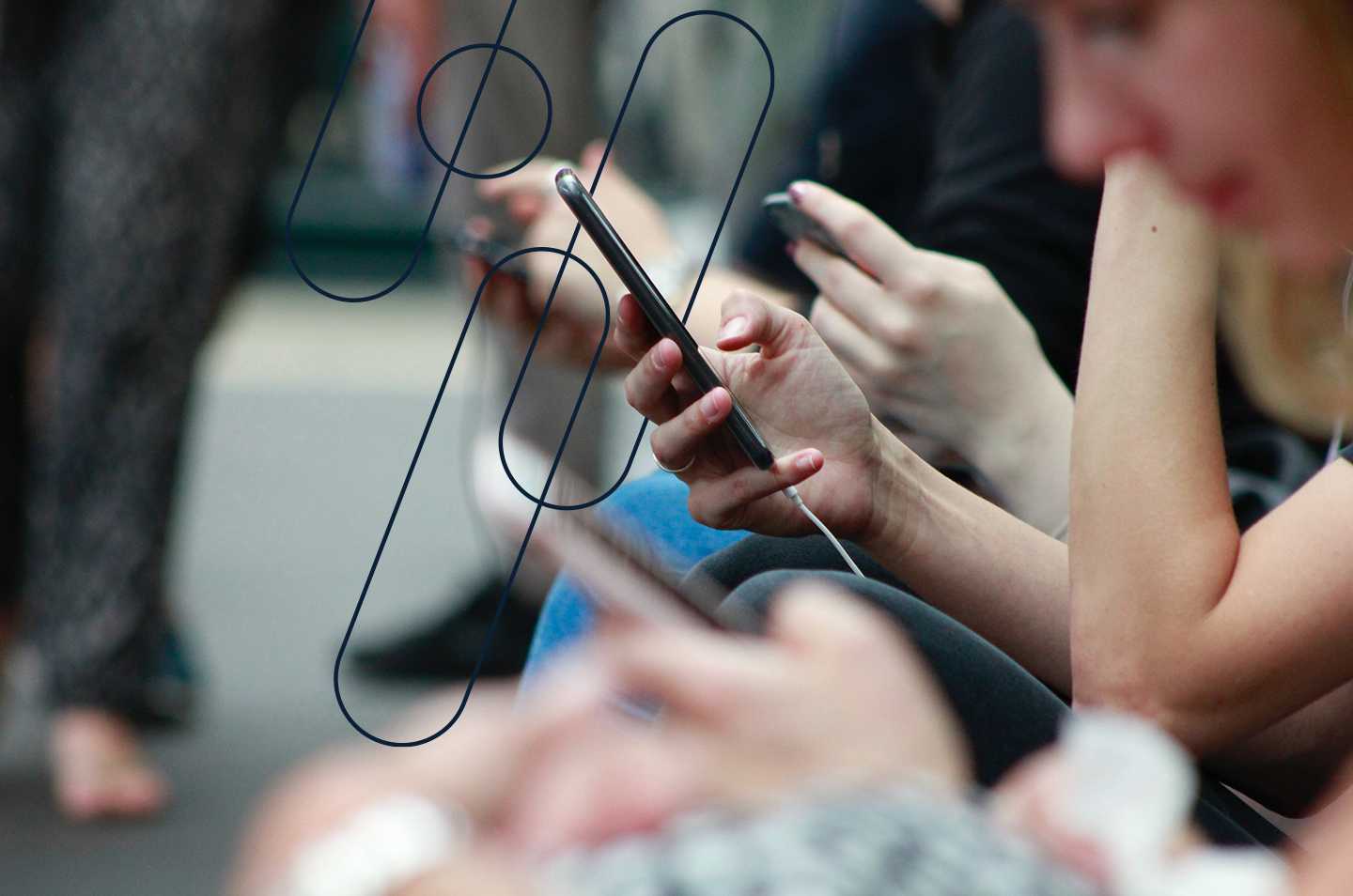
Data science—the broad interdisciplinary field focused on extracting insights from data— draws on concepts and methods across mathematics, statistics, computer science, and domain expertise to analyze large, complex datasets. Among the main tools in the data science toolkit is Machine Learning (ML), which refers to algorithms that can automatically learn patterns from data and use them to make predictions or decisions without explicit programming.
Another concept which is closely tied to both data science and machine learning, Natural Language Processing (NLP), focuses specifically on text-based data and language. The goal of NLP is to teach computers to understand, interpret, and manipulate human language. This supports a wide variety of applications, from search engines and chatbots to product recommendation systems and more. While NLP relies heavily on machine learning methods under the hood, it also requires an understanding of the way human languages are structured and convey meaning.
While data science encompasses a wide range of disciplines, it is important to note that machine learning, unlike NLP, specifically focuses on predictive modeling capabilities. NLP, on the other hand, utilizes these capabilities to extract valuable insights from human language data. But NLP also requires specialized knowledge and techniques to deal with the nuances of linguistic communication that standard machine learning algorithms may not account for on their own. Together, machine learning and NLP empower organizations to uncover data-driven insights from both numbers and unstructured text at an unprecedented scale. With the rising importance of user-generated content across social media, consumer reviews, support tickets, and more, NLP is becoming an increasingly crucial area of focus for business analytics.
Download the full whitepaper to learn more.